Data Science in Detail: Diego Hernán Díaz Martínez
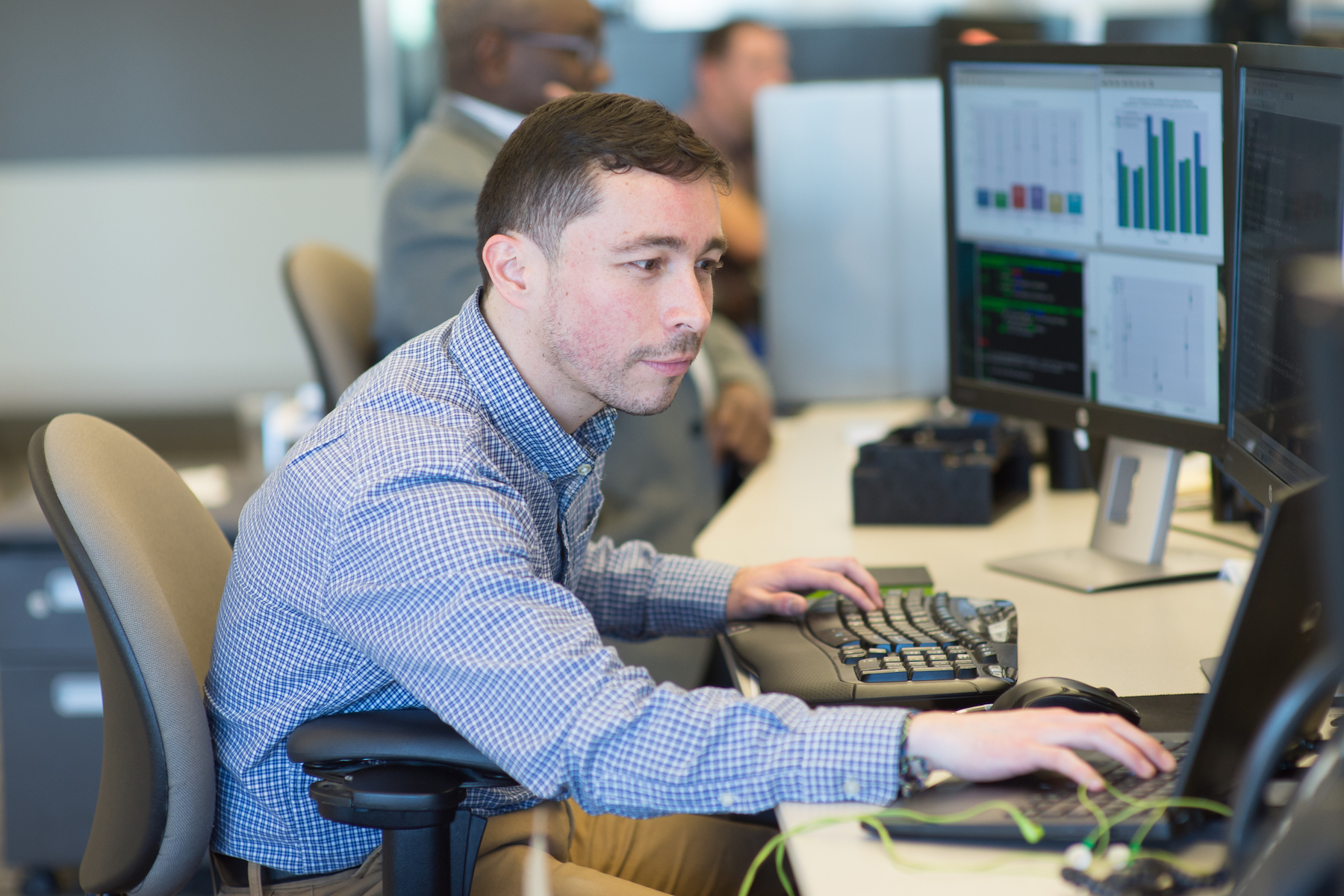
Florida State University alumnus Diego Hernán Díaz Martínez. Photo courtesy Clarios.
Diego Hernán Díaz Martínez graduated in 2016 with a master’s in biomathematics and a Ph.D. in mathematics from the Florida State University Department of Mathematics, part of the College of Arts and Sciences. After graduating, Diego accepted a position in the data science field as a battery design tools and analysis senior engineer at Clarios, a global energy storage solutions company.
Where are you from? What brought you to FSU?
I'm from Bogota, Colombia. I did my undergrad in mathematics and worked for three years as an actuary in risk management for a bank in Bogota. I decided to come to FSU because I felt the need to expand my knowledge in mathematics. The mathematics Ph.D. program at FSU is well renowned and its professors had interesting research topics going on, so I decided to apply and got accepted.
What inspired you to choose your major and specific area of research?
I had been using traditional mathematical tools during my time in the bank, but now I wanted to develop new mathematical methods that could be used in other real-life applications. Professor Washington Mio was working on very interesting projects related to shape analysis, geometry, and topology and had access to data coming from biological and medical experiments. These areas were new and exciting for me and aligned perfectly with my goals. Professor Mio gave me the opportunity to work with him at his lab, so I began my research on spectral geometry applied to statistics.
You currently work as a Battery Design Tools and Analysis Senior Engineer at Clarios. What does your job entail?
At Clarios, I'm in charge of developing statistical, mathematical, and electrochemical tools/models for the analysis of lead-acid battery data related to its design, manufacturing, quality, and field life. These models are used to understand and quantify the influence of different factors on battery performance and forecasting failure, for example. Factors include design inputs, manufacturing process, and external factors such as the type of vehicle, temperature, or driving patterns. Work ranges from creating simple scripts to analyze data and answer particular questions the organization has to developing stand-alone applications that other engineers can use.
What is your favorite thing about your job? What has been most surprising to you about the field of data science?
One of my favorite things about the job is the different types of data and projects the company has. One week I can be working on vehicle data captured via the car’s on-board diagnostic port, and the next one I can be working with data that comes from experiments done to batteries in the lab. This diversity in the projects has allowed me to learn and explore new methods and skills for the job.
What’s most surprising to me about the field of data science is that there are always real-life applications and problems that haven’t been approached using statistics or machine learning and that could benefit from these methods. There’s always space to create new things!
What advice do you have for students considering careers in data science?
My advice to all students considering careers in data science is to take their time to strengthen their foundations in mathematics and statistics. Mathematics are helpful not only in understanding the numerous machine-learning methods and knowing when and how to apply them, but are also important when trying to understand the physics that guide underlying processes that create the data for a particular application, which is useful in the design of relevant features. Statistics are important because not all tasks in data science are about prediction, but also about making inferences on the relationships between variables and accurately quantifying the impact some variables can have over others.